Data Collection and Processing
Our HumanShape™ online body shape models are based on accurate three-dimensional (3D) anthropometric measurements of people with a wide range of body characteristics, ages, and genders. Various state-of-the-art scanning systems, including VITUS XXL, 3D Systems Sens, were used to measure the 3D body contours and typical anthropometric dimensions. 3D digitizer, FaroArm, was also used to collect anatomical body landmarks, and these landmarks data were used to estimate kinematic joint locations. All the data were carefully collected and validated by our experienced research staff.
All the scan data were standardized by fitting a HumanShape™ template model. This template fitting process does not only facilitates analyzing the scan but also ensures anatomical homology across the scans. Our HumanShape™ model with 14k that is efficient to represent body shape was used as a template model. Vertex density in each body segment were optimized to express the details of segment geometry in the most efficient way. A two-level fitting method proposed in our previous study was used to fit the template (Park and Reed, 2015). Briefly, this method first morphs a template model based on the landmarks of the target scan using a radial basis function (RBF) technique. The initial morphing step ensures specific template vertices are located at corresponding landmark locations across all target scans. In a second step, an implicit surface fitting technique fits the morphed template to a target scan and captures geometric detail.
Statistical Analysis
A principal component analysis (PCA) was conducted using the methods described in Reed and Parkinson (2008). In brief, the coordinates of the template model vertices are flattened to create a geometry vector for each template fit . A set of standard anthropometric values were appended to each vector along with the 3D coordinates of the body landmarks and estimated joint center locations. PCA was conducted on these geometry matrices to find the linear projection that reduces dimension of the matrix while variance in the data is well preserved. The first 100 PC scores for the matrices were retained from the PCA in general for oue models.
Following the methods described in Reed and Parkinson (2008), a linear regression analysis was conducted to associate the projected PC scores with subject characteristic, e.g., stature, body mass index (body mass in kilograms divided by stature in meters squared), and the ratio of erect sitting height to stature (SHS) as predictors. For a more detailed description of the method, please find the related papers listed below.
Subject Pools
Select model type on the left to see subject pool
Child Model Subject Pool
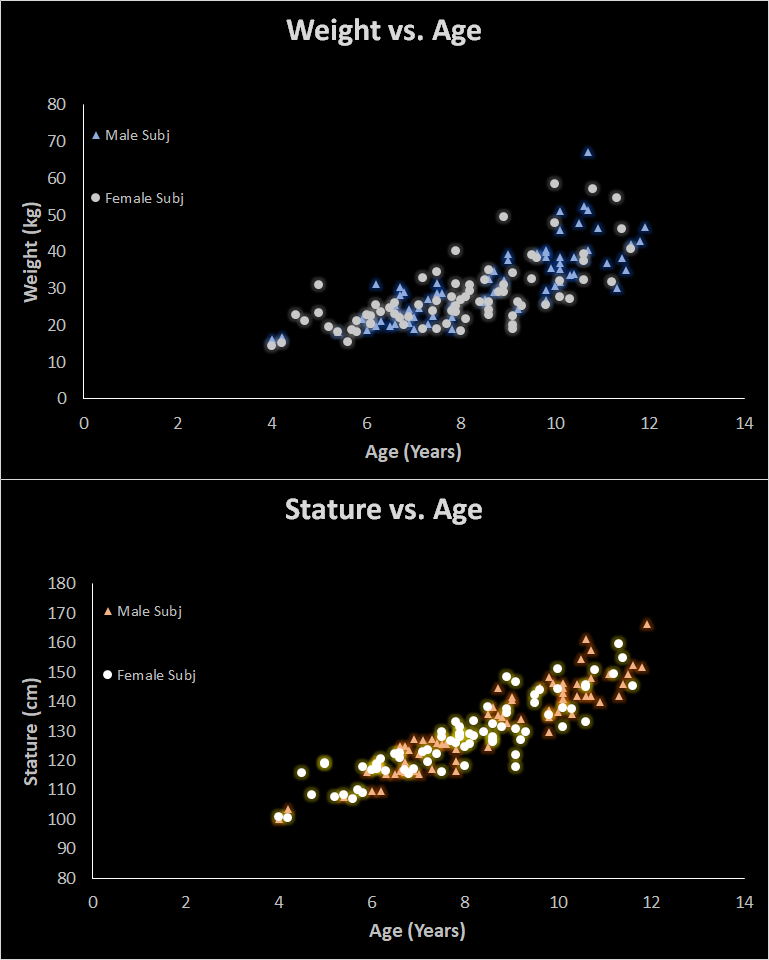
APPLICATIONS
The statistcal body shape model presented here is broadly applicable and can be readily extended to a wide range of applications. We have applied the model to fitting low-resolution data from Microsoft Kinect, demonstrating the rapid generation of avatars from Kinect data along with accurate prediction of standard anthropometry (Park and Reed 2014, Park et al. 2015). We expect that the model will be useful for generating anthropometric specifications for human surrogates used for safety applications, such as crash test dummies and finite-element models used for crash simulation. For these applications, the desired body size is usually specified using only a few variables, typically stature, body weight, and erect sitting height. We expect the model to be integrated into commercial ergonomics software, such as the Jack human modeling software, in the same manner as we have demonstrated using adult SBSMs (Reed et al. 2014). We also anticipate that this or similar SBSMs may be useful for stochastic investigation of the performance of protective equipment, which currently is performed using a small number of body forms. Likewise, clothing fitting simulations might be enhanced by the availability of a well-validated parametric model.
RELATED ARTICLES
-
Reed, M. P., & Parkinson, M. B. (2008). Modeling variability in torso shape for chair and seat design. In ASME 2008 International Design Engineering Technical Conferences and Computers and Information in Engineering Conference (pp. 561-569). American Society of Mechanical Engineers.
-
Park, B-K, Lumeng, J.C., Lumeng, C.N., Ebert, S.M., and Reed, M.P. (2014). Child body shape measurement using depth cameras and a statistical body shape model. Ergonomics, 58(2):301-309. 10.1080/00140139.2014.965754.
-
Park, B-K. and Reed, M.P. (2014). Rapid generation of custom avatars using depth cameras. Proc. 3rd International Digital Human Modeling Conference. Tokyo, Japan.
-
Reed, M.P., Raschke, U., Tirumali, R., and Parkinson, M.B. (2014). Developing and implementing parametric human body shape models in ergonomics software. Proc. 3rd International Digital Human Modeling Conference. Tokyo, Japan.
-
Park, B-K D., Ebert, S., and Reed, M.P. (2017). A parametric model of child body shape in seated postures. Traffic Injury Prevention. 18(5):533-536. 10.1080/15389588.2016.1269173